Understanding Data-platform Needs to Support Network Observability
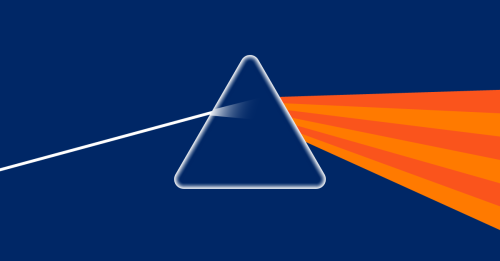
What is a data platform?What are the main features of a modern data platform?Data platform ingestionData platform storageData transformationData platform modelingData platform discoveryData platform observabilityData platform securityBusiness intelligence with data platformsWhat are the advantages of a data platform?What is network observability?What are the advantages of network observability?Supporting network observability with a unified data platform
Summary
By providing a central, single source of truth, a unified data platform reduces the risk of miscommunication for large, complex networks. In this article, we dive into how data observability can play a critical part in network observability.
In today’s digital world, data is generated faster and at a larger scale than ever before. With software architectures increasingly adopting distributed, cloud-based models, network infrastructures have become complex webs of virtual and physical devices.
The interplay of multiple, simultaneous deployments (multi-cloud, blue/green, canary), service-oriented production and infrastructure models, the ephemeral nature of the data associated with technologies like containers and serverless, thousands of data sources and destinations, and the need to monitor and act on all of this information, creates a high stakes environment for data management.
What is a data platform?
A data platform is an abstraction that offers a single pane of glass for managing your data wherever that data is being generated, processed, or stored.
Through instrumentation, integrations, automated analysis, visualizations, and a full suite of data management features, data platforms offer data managers and engineers a unique opportunity to interact with distributed data at a scale that would otherwise exist in siloed data infrastructures.
What are the main features of a modern data platform?
Data platforms offer enterprises a range of features:
- Data ingestion
- Data storage
- Data transformation
- Data modeling
- Data discovery
- Data observability
- Data security
- Business intelligence
Data platform ingestion
Useful data is generated at every layer of an application. Different formats, models, and protocols constrain data from these different domains accordingly. The act of incorporating this disparate data into a single, accessible data mass is called data ingestion.
In a modern network, data ingestion is likely to happen at multiple points, as some data needs to be sampled, analyzed, or otherwise processed before reaching the central data store/lake/warehouse.
Engineers build data pipelines for specific sources and destinations to structure incoming data for querying and subsequent analysis. Whether directly or through integrations, data platforms provide the tools needed to build and optimize these data pipelines. These pipelines provide a standard method of synthesis, which can be replicated across as many servers as it takes to ingest all of the data, enabling the rest of the system to work at scale across multiple teams for analytics.
Data platform storage
Data platforms offer a central interface for accessing, querying against, and managing the data storage services in your network, across infrastructure boundaries like cloud providers.
With storage metadata, data platforms offer engineers opportunities for additional insights into data access, resource allotment and capacity, performance, and more.
Data transformation
As data is ingested or moved between services, its values, structure, or format may need to be transformed to be consumable by its destination. To this end, data transformation may involve validating, scrubbing, and reconfiguring data. Some common data transformation techniques include data generalization, smoothing, aggregation, normalization, and the creation of novel data structures from ingested data (e.g., value1 * value2 = newDataPoint).
ETL (extract, transform, load) systems modify and coordinate data with other data streams. This is often the first point of data enrichment for network operators, providing an opportunity to correlate things like IP addresses with DNS names, application stack tags, and deployment versions.
Data platform modeling
Whether generating schemas and database profiles from abstract models or generating visualizations based on integrated data sources, an effective data platform will give engineers the data modeling tools they need to design, test, and better understand their data infrastructures.
Data platform discovery
One of the principal features of a data platform is providing a single, central location for cross-boundary data discovery. By synthesizing data from every part of your data infrastructure, data discovery enables pattern recognition and trend analysis impossible for human operators alone.
By being customizable and extensible, data platforms enable engineers to tailor their data discovery for various applications: dashboard creation, reporting, monitoring, cross-domain analytics, and more.
Data platform observability
Observability is the degree to which the internal state of a system can be deduced from its outputs. In distributed, hybrid cloud networks, data observability leverages information like logs, metrics, traces, and flow data to provide end-to-end visibility into the data lifecycle.
With instrumentation, contexts such as business domain, customer data, geolocation, feature flags, and blue/green deployments (really whatever proves to be useful) can be added to data as it moves through your networks. This context adds dimensionality to querying and modeling and gives operators and engineers a much better view of end-user experience.
A modern data platform will offer tools and interfaces for making the most from your observable data, including anomaly detection, root cause analysis, and highly-nuanced data discovery.
Data platform security
As modern SaaS solutions, data platforms offer organizations with highly complex data infrastructures the ability to manage access, craft and enforce security protocols, perform comprehensive threat analysis, and automate alerting and mitigation efforts.
Business intelligence with data platforms
The context of this data innovation is simple: driving business goals forward and delighting customers.
Data platform features like data observability, modeling, and discovery enable the next generation of business intelligence efforts. Offering unparalleled insight into customer behavior, logistics, and the economic performance of your data infrastructures, the ability to synthesize “big data” into novel and competitive insights is a crucial feature of data platforms.
What are the advantages of a data platform?
Here are the key advantages of a data platform:
- Centralized. By providing an abstraction that encompasses an enterprise’s data infrastructure, data platforms tear down data silos and give teams a single pane of glass for their data management.
- Comprehensive. From data creation to destruction and everywhere in between, data platforms provide the context and visibility to get the most from your data wherever it is in its lifecycle.
- Customizable. No two enterprises have identical data needs. Data platform features are highly customizable: visualizations, queries, and database management policies can all be fine-tuned from one service.
- Extensible. Data platforms allow organizations to add features and build novel services incorporating the full scope of an organization’s data.
- Scalable. Built to handle planet-scale operations, data platforms are designed to provide dynamic, scalable solutions across the data lifecycle.
- Secure. By simplifying access, facilitating strategies like microsegmentation, and providing real-time threat analysis that incorporates all your data sources, data platforms offer unique security management, alerting, and mitigation capabilities.
What is network observability?
Similar to data observability, network observability applies the notion that context is critical. In combination with the logs, metrics, trace, and flow data, network observability incorporates device (virtual or physical) data with the rich contextualization made available through instrumentation.
This context allows engineers and operators to “ask anything” and figure out the unknown unknowns in their networks. Network observability’s big data approach helps teams transcend simply monitoring their networks and makes the most of machine learning and automation to identify and mitigate performance and security issues.
What are the advantages of network observability?
Network observability’s unique approach offers distinct advantages to network operators:
- Visibility into all your networks
- Context
- Customizable queries
- Visualizations
- Real-time analysis
- Automated insights and solutions
Supporting network observability with a unified data platform
By providing a central, single source of truth, a unified data platform reduces the risk of miscommunication. This is particularly important in large, complex networks where different teams are responsible for various aspects of network operations.
Unified data platforms also facilitate using machine learning and artificial intelligence algorithms to detect and alert on network issues automatically. Although the utility of AI and ML in NetOps is emerging, having access to a unified data platform gives these technologies richer datasets.
In short, data platforms make the “big data” analysis central to network observability a possibility.
To see what network observability can do for you, get a Kentik demo today.