Data Enrichment Will Be the New Correlation
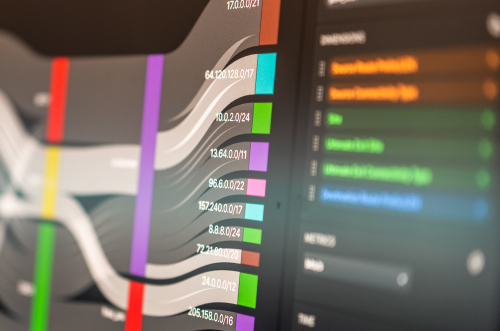
Summary
At one point, data was called “the new oil.” While that’s certainly an apt description for the insights we can extract from data, most organizations today are finding that new data repositories and “data lakes” often don’t provide the expected benefits due to the analytics challenge. CTO Jonah Kowall explains how advanced data enrichment techniques, leveraging AIOps technologies, can make the promise of data analysis a reality.
At one point, data was called “the new oil.” While that’s certainly an apt description for the insights we can extract from data, most organizations today are finding that new data repositories and “data lakes” often don’t provide the expected benefits due to the analytics challenge.
In fact, Gartner stated as a recent prediction that, “through 2022, only 20% of analytic insights will deliver business outcomes.” What this means is that—although we collect and store data—it’s what we do (or are not yet able to do) with it that counts.
When this idea is applied to operational tooling, the reality is far worse. We’ve been touting the linkages between development, testing, operations, and automation for decades. However, the reality is that we’ve only begun to solve part of the problem. The closed-loop nature of monitoring and automation is still only being addressed in modern companies who develop a lot of custom automation code.
In the traditional enterprise, where systems are highly variable and have different ages, the reality is different.
Gartner has coined the term “AIOps” to advance these techniques by applying machine learning (ML) and artificial intelligence (AI) to the problem in order to better address the challenge. They point out three main areas where AIOps can be applied, which I’ll dig into further in this blog post:
- Root Cause Analysis (RCA)
- Log Analytics
- Event Correlation
Root Cause Analysis
From Gartner’s list, root cause analysis (RCA) can benefit most from the application of AIOps techniques. This is what is occurring in the APM arena where guided root cause is becoming more of a common feature across market-leading products such as AppDynamics, Dynatrace, New Relic, and upstart Instana.
These APM tools have their own data collection mechanisms in the form of software agents and other proprietary technologies. Granted, there are open-source agents and APIs out there, but they are largely not used for the RCA process as they lack the depth or context of these proprietary software agents.
These tools face future challenges as the diversity of data sources increases: Their need to control the input into their algorithms and requirement to continually build new agents and instrumentation are a losing battle. That’s why there are so many emerging standards in APM for agents, APIs, and the way data is collected.
All of these are doomed before they begin due to the diversity of and variance in the applications, languages, and frameworks. This is a futile exercise, but that doesn’t mean companies won’t spend billions of dollars trying to solve it.
Log Analytics
When it comes to creating insights out of data not generated by a monitoring system, log analytics immediately comes to mind. All infrastructure technologies and custom applications generate logs without any standard semantics as to what these messages mean and the importance of the messages themselves.
Applying ML and AI techniques to this data will result in great gains in productivity by users and other systems. We’ve seen Splunk, Elastic, and Sumo Logic investing heavily in moving this market forward and embracing the application of these new analytics to logs.
These techniques, while they improve the type of data you can extract from difficult-to-understand logs, still lack details around the relationships between data, such as where specific logs come from or how they relate back to a specific transaction or user. Thus, they’re great tools for basic or advanced troubleshooting, but not much else.
Some of the log companies have almost evolved to building more advanced workflows and use cases similar to event correlation.
Event Correlation
As we’ve seen evolution in other areas, the application of ML and AI has been the most dramatic in event correlation. These systems generally consume more structured, event-based data from other monitoring systems. Their goal is to extract what is important and what is not and determine how the events are related.
While these tools often deal with challenges similar to log management systems, event correlation tools have a major advantage in that the problem space is smaller, more widely known, and better controlled in terms of data inputs. This has enabled innovators such as Moogsoft and BigPanda to create distinct advantages from legacy technology providers who once owned this area and domain.
All is good in AIOps… or maybe not, since we still have major pain even amidst the gains. The issue with event correlation systems is that they ingest and analyze data, and the data behind the correlation decisions is discarded or summarized to make the problem more easily solved at scale. The log analytics solutions have an entirely different problem where they do not correlate until query time, resulting in very slow performance at scale. Most of these solutions also require that the data being correlated is well-defined and either adds new data, or creates additional meaning, during ingestion.
Multidimensional Data Enrichment
One of the key areas not being addressed in current approaches is that correlation is not just for analyzing monitoring events. Correlation can also be done inside of monitoring systems. This is something Gartner alludes to in its research, but there are generally very few examples offered.
Data enrichment is something that needs to be done to provide additional context to existing data, using completely different data sources. This is often done in a primitive manner: Pulling in metadata such as tags (e.g., from a cloud platform) or orchestration engines (e.g., Kubernetes) in order to provide more context around metrics. However, it’s never done in a multidimensional way at scale.
The ability to overlay multiple data sets—including orchestration, public cloud infrastructure, network path, CDNs involved in the delivery of traffic… even security and threat-related data—is essential to create the additional context needed for algorithms to provide better insights.
There are very few platforms that can accomplish this type of enrichment at scale, not only due to the data storage challenges, but also because most simply lack the ability to ingest and correlate data in this manner.
I was not aware of these types of capabilities before joining Kentik, but the company has been building and executing this type of multidimensional enrichment, at scale, for the last several years—all in an effort to create a next-generation AIOps platform for the network professional. Ingesting and correlating data to create additional context is required to create the level of situational awareness that lets network professionals make the right decisions, every time.
As we evolve Kentik’s data platform and capabilities we will be rethinking what is possible in order to bring the most scalable and capable platform to market.
To be the first to know about our latest developments, subscribe to the blog.
Related Reading: