The Role of Predictive Analytics in Network Performance Monitoring

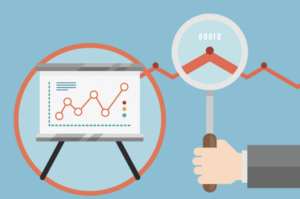
Summary
Predictive analytics has improved over the past few years, benefiting from advances in AI and related fields. In this post, we look at how predictive analytics can be used to help network operations. We also dig into the limitations and how the accuracy of the predictions depends heavily on the quality the data collected.
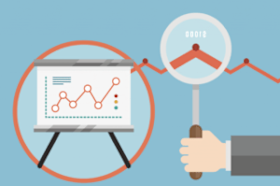
Predictive analytics uses historical data to predict future events. In the context of IT practices, the analytics are gaining in interest, in part, due to advances in supporting technologies, such as big data, machine learning, and artificial intelligence. For network performance monitoring (NPM), predictive analytics can be used to help network operations teams identify potential network failures and performance issues with greater accuracy and lower mean time to repair (MTTR). To address issues before they affect network operations, key performance metrics are monitored and analyzed. Identifiable patterns are then used to facilitate network changes to deal with performance issues or possible security threats.
Key Use Cases for NPM
- Determining network performance — Machine learning can be used by predictive analysis for network performance optimization. By predicting capacity problems accurately, operations teams can act preemptively to rebalance the load on a network and provision the network with more capacity. Predictive analytics can also examine trends in data traffic patterns based on usage type and provide an early warning whenever it discovers possible issues.
- Identifying security threats — Predictive analytics enables security analysis to recognize anomalous behavior from systems, devices and/or users. Rapid detection of security breaches are more important than ever, and predictive analytics can provide clues that escape human observers. Predictive analytics, along with NetFlow (or other flow data variants), can help weigh the risk of devices on a network and predict which are at highest risk. The cost of a network breach is typically several million dollars, so the more quickly you can detect and correct the breach, the less cost and impact there will be to your company’s reputation and their bottom line.
The Caveat
As network operators implement network function virtualization (NFV) and software-defined networks (SDN) methodologies, operations teams will be dealing with a higher frequency of network changes. That makes it harder to predict network performance or security anomalies. With constant network changes becoming the norm, historical data may also be less available making predictions less accurate.
Big Data and Cloud-Scale Resources to the Rescue
Even with sophisticated predictive learning capabilities, the level of prediction accuracy still depends on the quality, detail, and accessibility of the data set.
With all this data, network performance monitoring tools are necessary to extract insights and trends. Machine learning techniques are used to find patterns in data and to build models that predict future outcomes. A variety of machine learning algorithms are available, including linear and nonlinear regression, neural networks, support vector machines, decision trees, and other algorithms.
Advancements in distributed storage have opened the way for big data to play a pivotal role in providing a foundational platform for predictive network analytics. And while most network operators have invested in big data repositories, they need to be designed at the outset not as an afterthought to address predictive analytics use cases. Use case-driven predictive analytics is key to effectively storing and using all of this data.
The availability of cloud-scale compute power has paved the way for advanced predictive network analytics algorithms. These algorithms require immense compute power to implement machine learning techniques in near real-time that combine and contextualize all of this data.
Predictive analytics has improved over the past few years, benefiting from advances in AI and related fields. The limitation of AI is that the accuracy of the predictions depends heavily on the quality the data collected.
A big data, SaaS approach is ideal for unifying network data at scale and providing the compute power to achieve predictive analytics benefits. If you’re interested in learning more about how Kentik’s big data approach is used for predictive analytics, check out the Kentik Detect for DDoS Protection solution brief. If you already know that you want to implement a much more cost-effective network security and planning solution for your institutional network, start a free trial.